New Google algorithm makes robots better equipped at recognizing transparent objects
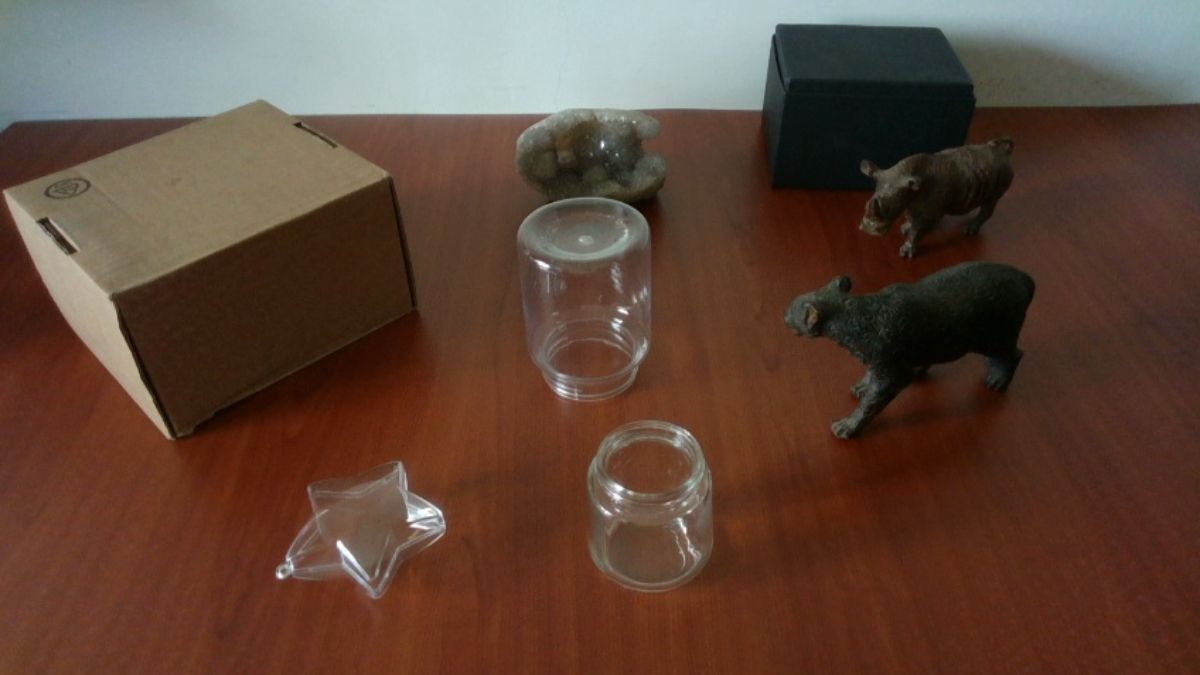
ClearGrasp, a new learning algorithm has been developed by researchers at Google Synthesis AI, and Columbia University, which will help robots interact with transparent objects. The algorithm uses RGB-D images to recreate the 3D spatial information of the object in question.
Robots make use of the RGB-D cameras to paint an accurate 3D picture of the environment that it is in. However, there certainly are limitations to the surroundings created by such cameras; for example, it does not work effectively for transparent objects such as glass.
To recreate 3D spatial information for transparent objects proved itself to be a herculean task for the researchers. There was very little data available meant for transparent surfaces, and most of the data blatantly ignored the transparent surfaces. To overcome this issue, the researchers created a large-scale transparent object data set, containing 50,000 realistic renderings of various object surfaces.
The ClearGrasp algorithm uses 3 neural networks to correctly identify transparent objects. One of the networks estimates the surface normal vector, one of them calculates the edge of the occlusion and the other one calculates the transparency of the object. The mask of the object is used to exclude pixels of non-transparent objects in order to fill the correct depth.
The global optimization module can predict the normal vectors of other surfaces from surfaces of known depth to reconstruct the shape of the object and to differentiate between two objects.
However, the algorithm could not correctly detect the normal vectors of other basic surfaces, due to the limitation of the synthetic data set. To tackle this problem, the researchers came up with the Matterport3D and ScanNet data set.
However, all of these mishaps aside, ClearGrasp is the only algorithm available that can reconstruct the depth of transparent objects, increasing the success rate of grasping transparent objects by the robotic arm from 12% to 74%.
No comments